Using Artificial Intelligence to Improve the Safety of Gallbladder Surgery
Laparoscopic cholecystectomy, or the removal of the gallbladder via keyhole surgery, is one of the most commonly performed general surgery operations with over 40,000 performed each year in Australia. The operation carries with it a small but significant risk of injury to the bile duct, a tube that carries bile from the liver and gallbladder to the intestine. This complication has major consequences a significant increase to health-related expenditure, income loss, increased morbidity and reduced life expectancy.
Artificial intelligence (AI) is the computer simulation of intelligent behaviour and critical thinking and the performance of complex cognitive tasks such as object and word recognition, decision-making and learning. AI and machine learning has growing application in medicine and surgery and has the potential to improve surgical outcomes.
We hypothesise that the use of machine learning in laparoscopic cholecystectomy can improve surgical safety and reduce BDI through improved anatomical structure identification, the recognition of unsafe lines of dissection, early recognition of BDI, automatic and effective documentation of the critical view of safety and through training and performance assessment. This project is in partnership with the St Vincent's Hospital upper GI research unit, led by Mr Matthew Read in conjunction with the University of Melbourne Department of Biomedical Engineering with co-investigators Dr Catherine Davey and Prof James Bailey.
Impact
Machine learning technology has the potential to revolutionise surgical practice with many applications from augmented reality, decision support and advanced surgical training. This work will make a significant contribution to what is a rapidly growing and exciting field.
Current Stage
We are currently in the data collection and data labelling phase of the project. We aim to collect and label 100 videos of laparoscopic cholecystectomy. With this data, we will train and validate a convolutional neural network (CNN) that is capable of autonomously recognising anatomy and surgical instruments.
We have successfully trained a CNN on a limited dataset from a single video that is capable of recognising anatomy and instruments on unseen frames (see image below). With a more extensive dataset, we expect to be able to improve our accuracy and generalisability. On completion of labelling and training, we will run a pilot study to demonstrate the clinical use and effectiveness of the technology.
Preliminary image data demonstrating computer generated structure labelling superimposed onto the surgical field. Each colour represents a different structure.
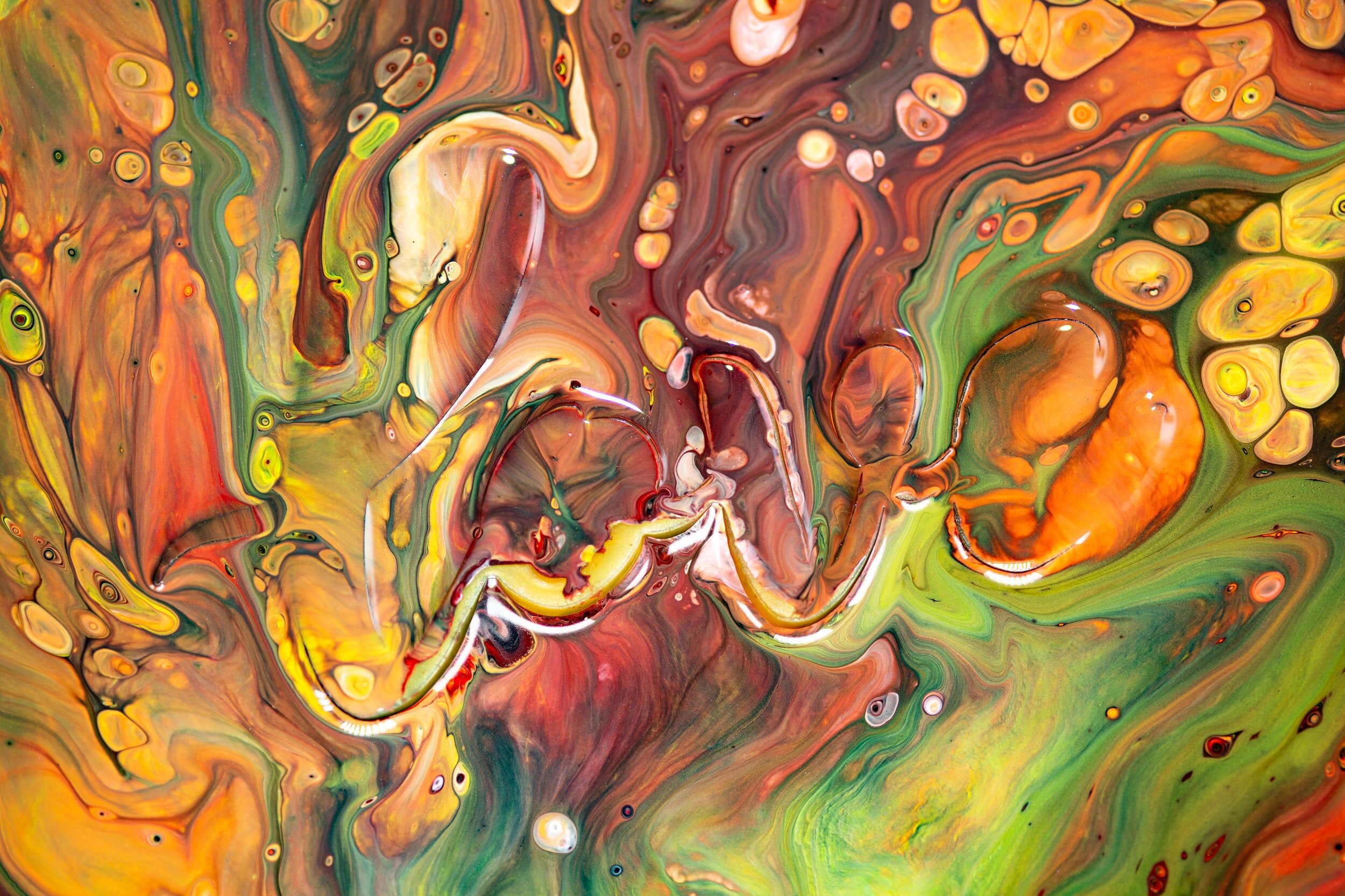
Status: Currently recruiting
Site: St Vincent’s Hospital Melbourne
The University of Melbourne
Ethics: HREC/67934/SVHM-2020-235987
Principal Investigator: Mr Matthew Read
Eligibility
Inclusion
Adults ≥18 years of age
Undergoing elective laparoscopic cholecystectomy
Inclusion
Unable to provide own consent
Outcome Measures